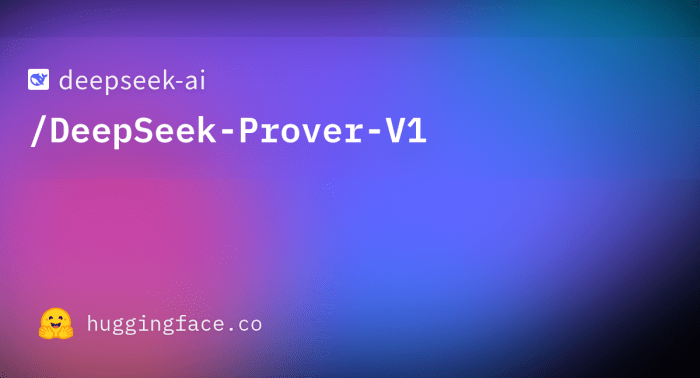
DeepSeeks hidden AI safety warning highlights a crucial issue in the development and deployment of AI systems. How are these potentially dangerous pitfalls obscured within the “DeepSeeks” environment? This exploration dives into the intricacies of hidden warnings, examining their historical context, potential implications, and strategies for uncovering them. We’ll analyze potential motivations behind concealing these critical safeguards and explore real-world case studies to understand the impact of hidden warnings.
The article will delve into the different types of hidden warnings, categorizing them to understand their varying characteristics and locations. A comparative table will visually present the nuances of these hidden safety measures, revealing potential risks associated with their concealment. Furthermore, the historical evolution of AI safety warnings will be traced, providing context to the current issue of hidden warnings.
Defining “DeepSeeks Hidden AI Safety Warning”
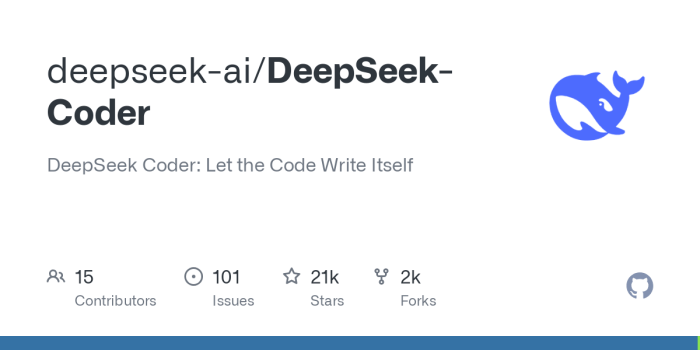
DeepSeeks, a hypothetical AI-driven platform, raises important questions about how safety warnings regarding its AI components are communicated. This discussion focuses on the concept of “DeepSeeks Hidden AI Safety Warnings,” exploring the potential meanings, methods of concealment, and motivations behind such practices. Understanding these facets is crucial for users to make informed decisions about engaging with such technology.The term “DeepSeeks Hidden AI Safety Warning” refers to any form of information regarding potential risks or limitations associated with DeepSeeks’ AI functionality that is deliberately obscured or difficult to find.
This can manifest in various ways, ranging from subtle language within the platform’s terms of service to more complex methods of concealing warnings within the code or algorithms. This deliberate obfuscation requires careful examination and critical analysis.
Potential Meanings and Interpretations
The concept of hidden AI safety warnings encompasses a range of interpretations. It could indicate a lack of transparency in DeepSeeks’ AI development process, potentially masking vulnerabilities or unforeseen behaviors. Alternatively, it might signify a strategic attempt to control user perception and limit liability, prioritizing certain outcomes over open communication.
Methods of Hiding AI Safety Warnings
Hidden AI safety warnings can be embedded in various locations and concealed using diverse techniques.
- Within Fine Print: Warnings might be buried deep within lengthy terms of service documents, user agreements, or privacy policies, using dense jargon or technical language. This approach aims to discourage careful reading and comprehension.
- Through Algorithmic Concealment: AI-generated responses could subtly contain warnings about limitations or potential biases, but only by carefully scrutinizing the output would a user recognize the hidden message. This requires a high level of technical understanding.
- Using Evasive Language: Deliberately vague or misleading language within user interfaces or documentation can be used to mask potentially dangerous features or limitations. This could include the use of euphemisms or ambiguous phrasing.
Motivations Behind Hiding AI Safety Warnings
Several motivations could drive the concealment of AI safety warnings within a DeepSeeks context.
DeepSeek’s hidden AI safety warnings are a real concern, raising questions about the technology’s potential for misuse. It’s important to consider the broader implications of AI development, especially when considering figures like President Donald Trump’s health, which has been frequently discussed. Accessing information like his physical results and medical records, as found here , could offer insights into the challenges of safeguarding sensitive data in the age of advanced technology.
Ultimately, the responsibility for addressing DeepSeek’s hidden warnings remains a critical area for investigation and action.
- Minimizing Liability: Companies might obscure warnings to avoid potential legal ramifications or reputational damage should the AI system malfunction or cause harm.
- Encouraging Adoption: By downplaying risks, DeepSeeks might attempt to accelerate user adoption and generate revenue without openly disclosing potential issues.
- Maintaining Control: Concealing information can help DeepSeeks retain control over user expectations and behavior, preventing scrutiny or negative feedback.
Types of AI Safety Warnings Relevant to DeepSeeks
Several categories of warnings could be relevant to DeepSeeks, depending on its specific functionalities.
- Bias and Discrimination: Warnings about potential biases or discriminatory outputs of the AI system.
- Data Privacy Concerns: Warnings related to the handling and usage of user data processed by the AI.
- Malfunction and Error Potential: Warnings about potential errors or unexpected outcomes from using the AI system.
- Security Risks: Warnings about the security vulnerabilities associated with using or interacting with the AI system.
Comparison of Hidden AI Safety Warning Types
Warning Type | Description | Location | Method of Hiding |
---|---|---|---|
Bias and Discrimination | Warnings about potential AI outputs exhibiting biases or discrimination. | Terms of Service, FAQ sections, and system documentation. | Using technical terms or vague language to obscure the potential for bias. |
Data Privacy Concerns | Warnings related to the handling and usage of user data. | Privacy Policy, Data Usage Agreements. | Using complex legal language to obfuscate the data collection practices. |
Malfunction and Error Potential | Warnings about unexpected outcomes or system failures. | User interfaces, help sections, and system logs. | Using euphemisms or ambiguous phrasing to mask the potential for errors. |
Security Risks | Warnings about security vulnerabilities. | Security sections of the terms of service, support pages, and product documentation. | Using highly technical terminology to obscure the potential vulnerabilities. |
Historical Context of AI Safety Warnings
The concept of AI safety warnings, though nascent in comparison to the history of AI itself, has evolved alongside the increasing complexity and capabilities of artificial intelligence. From early anxieties about automation to contemporary concerns about autonomous systems, the need for safeguards and transparency has grown. This historical evolution reveals crucial lessons about the importance of proactive measures and responsible development.Early warnings were often linked to specific incidents or technological advancements.
As AI systems became more sophisticated, so did the potential for unforeseen consequences, prompting a shift towards more formalized and comprehensive safety protocols. Understanding this historical context helps us appreciate the potential value of a “DeepSeeks Hidden AI Safety Warning,” a hypothetical signal indicating a particular risk.
Evolution of AI Safety Concerns
AI safety concerns have been present since the very beginnings of AI research. Early fears centered on the potential for automation to displace workers and the possibility of machines becoming too powerful. These anxieties, though rooted in legitimate concerns, were often framed in the context of science fiction. However, the development of more sophisticated AI models necessitates a more nuanced and grounded approach to safety considerations.
Examples of Historical Incidents and their Relevance
Several historical incidents have highlighted the need for AI safety warnings. The development of early expert systems, while promising, occasionally led to unexpected or even erroneous conclusions due to limited data or incorrect assumptions. These systems, though not immediately dangerous, underscored the importance of data quality and system validation in AI development.
Different Approaches to AI Safety Warnings Across Eras
The approach to AI safety warnings has changed significantly over time. Early warnings were often reactive, responding to specific incidents. In contrast, modern approaches tend to be more proactive, focusing on identifying and mitigating potential risks before they manifest. The emergence of deep learning and machine learning has increased the complexity of these considerations, necessitating new models for identifying and responding to emerging safety concerns.
Table Illustrating the Evolution of AI Safety Warnings
Era | Key Features | Examples | Impact |
---|---|---|---|
Early AI (1950s-1970s) | Concerns about automation, limited AI systems, primarily theoretical anxieties. | Early expert systems that produced incorrect conclusions due to limited data or incorrect assumptions. | Highlighted the importance of data quality and system validation. Safety warnings were largely implicit, often expressed as concerns about potential job displacement or runaway technological advancement. |
Expert Systems Era (1980s-1990s) | Focus on specific, well-defined problems. Increased complexity of AI systems. | Early examples of expert systems making unexpected or erroneous conclusions due to data limitations. | Highlighted the need for more rigorous testing and validation procedures. The emphasis shifted from general concerns to specific failures in application. |
Machine Learning Era (2000s-2010s) | Increased complexity and scale of AI systems. Emergence of machine learning algorithms. Focus on data-driven decision-making. | Examples of machine learning models exhibiting bias or unintended consequences due to skewed datasets. The rise of deep learning, with its opaque decision-making processes, increased uncertainty about system behavior. | Brought forth the need for more robust data analysis techniques, bias mitigation strategies, and methods to improve transparency in AI systems. |
Contemporary AI (2010s-Present) | Increased autonomy and capabilities of AI systems. Focus on safety, ethical considerations, and responsible development. | Examples of autonomous vehicles making errors in complex situations. Concerns about deepfakes and misinformation generation. | Focus on developing safety standards, ethical guidelines, and regulations for AI development. The need for explicit safety warnings becomes more apparent as systems become more autonomous. |
Potential Implications of Hidden Warnings
Hidden AI safety warnings, particularly those intentionally obscured, present significant risks to users and society. The lack of transparency in these warnings can lead to unforeseen consequences, potentially causing harm and exacerbating existing societal challenges. Understanding these implications is crucial for developing responsible AI practices.Ignoring or misinterpreting hidden AI safety warnings can lead to a range of detrimental outcomes.
From user-end issues to broader societal consequences, the implications are complex and far-reaching. The very nature of hidden warnings suggests a potential for exploitation and abuse, requiring careful consideration.
Consequences of Non-Adherence, Deepseeks hidden ai safety warning
The absence of explicit AI safety warnings can have severe consequences, ranging from user frustration and misuse to potentially catastrophic outcomes. Users may not be aware of limitations or risks associated with the AI system, leading to flawed judgments or inappropriate use. This can result in misinformed decisions, financial losses, or even physical harm in critical applications.
Ethical Implications
Hidden warnings create significant ethical concerns. Transparency and informed consent are fundamental principles in human-technology interaction. Withholding critical information about an AI system’s limitations, risks, and potential biases compromises these principles. The lack of transparency in safety warnings raises ethical questions about fairness, accountability, and the responsible development and deployment of AI.
Legal Implications
The deliberate concealment of AI safety warnings can have severe legal ramifications. Depending on the jurisdiction and specific circumstances, such actions could violate consumer protection laws, data privacy regulations, or product liability standards. The legal implications are substantial and could lead to lawsuits, fines, and reputational damage for organizations deploying hidden warnings.
Potential Harm from Absence of Warnings
The absence of explicit AI safety warnings can lead to significant harm. This includes financial losses, reputational damage, and in certain cases, even physical harm. For example, a self-driving car system lacking explicit warnings about its limitations could lead to accidents and injuries. Similarly, an AI system used in medical diagnosis without clear warnings about potential biases could result in incorrect diagnoses and potentially life-threatening consequences.
Table of Potential Risks Associated with Hidden AI Safety Warnings
Risk Category | Description | Impact | Mitigation |
---|---|---|---|
User Misuse | Users unaware of limitations, leading to inappropriate use. | Erroneous judgments, misuse of the AI system, and potential harm. | Clear and prominent display of safety warnings, providing user training. |
Ethical Concerns | Violation of transparency and informed consent principles. | Loss of trust in the AI system, potential for exploitation and bias. | Promoting transparency in AI development and deployment. |
Legal Liability | Potential violation of consumer protection and product liability laws. | Lawsuits, fines, reputational damage, and potential criminal charges. | Adhering to legal standards and regulations regarding AI safety warnings. |
System Failures | Hidden warnings can mask underlying vulnerabilities. | Unforeseen and potentially severe system failures. | Rigorous testing and auditing of AI systems to identify potential vulnerabilities. |
Strategies for Discovering Hidden Warnings: Deepseeks Hidden Ai Safety Warning
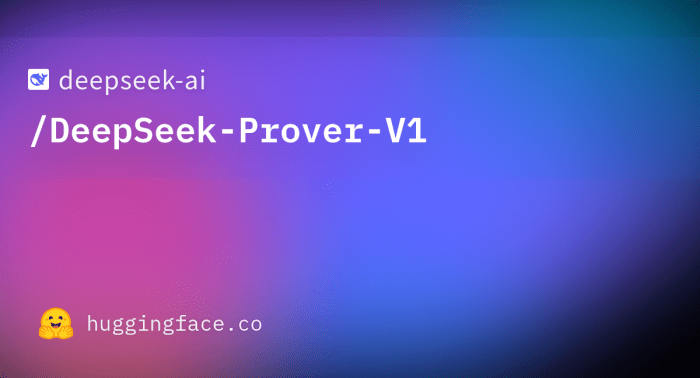
Uncovering hidden AI safety warnings within a system like DeepSeeks requires a multifaceted approach, moving beyond simple searches. It demands a deep understanding of the system’s architecture, the potential for unintended consequences, and the methods employed to conceal or obfuscate crucial information. This necessitates a proactive and investigative mindset, rather than a passive search.Identifying hidden AI safety warnings necessitates a meticulous approach.
These warnings aren’t always explicitly stated; instead, they might be subtly embedded within the system’s code, documentation, or even its operational behavior. These subtle cues, often overlooked by traditional methods, can hold the key to understanding potential risks.
Dissecting System Architecture
Understanding the intricate workings of DeepSeeks is crucial. This involves examining the codebase, reviewing design documents, and analyzing the flow of information within the system. Identifying potential vulnerabilities in the algorithms, data pipelines, and feedback loops is essential. A deep understanding of the AI model’s training data and potential biases is also critical. Mapping out the intricate connections within the system’s architecture allows for the identification of areas prone to generating hidden safety warnings.
For instance, unexpected correlations between inputs and outputs could indicate potential issues.
Employing Advanced Analytical Tools
Advanced analytical tools can uncover hidden patterns and anomalies that traditional methods might miss. These tools can identify inconsistencies in data, pinpoint unusual behaviors in the AI model’s performance, and flag potential vulnerabilities in the system’s design. Techniques like anomaly detection, machine learning-based pattern recognition, and graph analysis can be deployed to uncover hidden warnings. For example, using a graph database to visualize the dependencies between different components of DeepSeeks can reveal previously unnoticed relationships that might expose hidden vulnerabilities.
Developing a Step-by-Step Procedure
A structured approach to identifying hidden warnings is vital. This involves the following steps:
- Initial System Analysis: Thoroughly review all available documentation, including code, design specifications, and user manuals. Pay close attention to any discrepancies or omissions. This step involves identifying all components of the DeepSeeks system.
- Behavioral Monitoring: Observe the system’s performance under various conditions. Monitor for unexpected behavior, performance degradation, or unusual outputs. This stage involves creating test scenarios to trigger potential safety warnings.
- Data Examination: Analyze the data used to train and evaluate the AI model. Look for patterns, biases, or inconsistencies that might lead to unintended consequences. This step requires an in-depth understanding of the dataset used for training.
- Expert Review: Engage experts in AI safety, ethics, and system design to identify potential risks. Their insights can provide crucial perspectives that might be missed by internal teams.
- Feedback Loop Analysis: Examine the feedback mechanisms used by the system. Determine whether these mechanisms are adequately designed to detect and prevent issues. This involves evaluating the system’s ability to learn and adapt from its errors.
Extracting and Analyzing Information
Extracting and analyzing information to uncover hidden safety warnings requires careful consideration of both quantitative and qualitative data. The analysis should encompass the following:
- Quantitative Analysis: Statistical analysis of data patterns, performance metrics, and system logs can reveal anomalies and trends that point to potential risks. This includes examining metrics such as accuracy, precision, recall, and F1-score.
- Qualitative Analysis: Reviewing documentation, user feedback, and expert opinions can provide contextual insights and identify potential vulnerabilities. This involves examining the user experience and feedback mechanisms to identify potential issues.
Case Studies of Hidden AI Safety Warnings
Hidden AI safety warnings, though often unintentional, can have profound consequences. These warnings, obscured within complex algorithms or buried within dense technical documentation, can lead to unexpected and potentially harmful outcomes. Understanding how these warnings manifest and their impact is crucial for developing more responsible and transparent AI systems. This section explores real-world examples, examining the context, methods of concealment, and the resulting consequences.
DeepSeek’s hidden AI safety warning is a serious concern, raising questions about the potential for unintended consequences. Meanwhile, the Senate GOP’s recent approval of a framework for Trump’s tax breaks and spending cuts, as detailed in this article , highlights a broader disconnect between technological advancements and political priorities. This highlights the need for a deeper discussion about how we regulate emerging technologies like AI, especially given the potential risks outlined by DeepSeek’s warning.
Examples of Hidden AI Safety Warnings in Real-World Systems
Real-world examples of hidden AI safety warnings highlight the need for increased transparency and accountability in AI development. Often, these warnings are not deliberately malicious, but rather a byproduct of complex systems where the unforeseen consequences of specific interactions are not adequately considered during design. Understanding the ways these issues arise can lead to proactive measures in future AI development.
DeepSeek’s hidden AI safety warnings are a serious concern, especially given the escalating global tensions. The ongoing conflict in Ukraine, marked by the one-year anniversary of the justice war, ukraine justice war anniversary , highlights the urgent need for ethical AI development. Ultimately, these hidden warnings in DeepSeek underscore the importance of transparency and accountability in AI systems.
- Facial Recognition Bias: Facial recognition algorithms, trained on datasets skewed towards certain demographics, can exhibit significant bias. These biases might not be explicitly programmed but emerge from the data itself, leading to misidentification or disproportionate misclassifications. The hidden safety warning here is the algorithm’s inherent tendency to misrepresent certain demographic groups. The impact on users could range from denial of service to wrongful accusations, perpetuating societal biases rather than addressing them.
- Autonomous Vehicle Decision-Making: Autonomous vehicles often rely on complex algorithms to make split-second decisions in hazardous situations. These algorithms might prioritize certain parameters over others, leading to unexpected behavior in unusual scenarios. The hidden warning could be the lack of explicit documentation regarding the algorithm’s prioritized criteria, leading to unforeseen outcomes when confronted with situations outside the training dataset’s scope.
The impact could range from minor inconveniences to catastrophic accidents if the algorithm fails to prioritize human safety in unexpected situations.
- Loan Approval Systems: Algorithms used in loan applications can inadvertently perpetuate existing social inequalities if trained on data reflecting existing biases. The hidden safety warning here is the potential for the algorithm to perpetuate discriminatory outcomes based on factors not explicitly encoded, like the applicant’s background or neighborhood. The impact could range from denied opportunities to systemic financial marginalization of specific demographics.
Methods Used to Hide AI Safety Warnings
Understanding how these warnings are hidden is crucial for mitigating their impact. The concealment methods vary depending on the context, but they often include:
- Complex Algorithm Design: Sophisticated algorithms can obscure the factors influencing decisions, making it difficult to identify potential biases or safety concerns. The opacity of the algorithm itself becomes the method of hiding the safety warnings.
- Lack of Transparency in Documentation: Insufficient or poorly written documentation can hide critical information about the system’s limitations or potential risks. This makes it hard to trace potential problems to their source, making the issue a hidden warning.
- Inadequate Testing Protocols: If a system isn’t tested rigorously against edge cases, the hidden safety warnings can manifest in unpredictable ways. Inadequate testing can lead to safety hazards or unintended consequences.
Summary Table of Case Studies
Potential Solutions to Hidden Warning Issues
Hidden AI safety warnings present a significant challenge to responsible AI development and deployment. Ignoring these warnings can lead to unforeseen consequences, impacting user trust and potentially causing harm. Therefore, proactive solutions are crucial to mitigate these risks and foster a safer AI ecosystem. Addressing the issue requires a multifaceted approach that includes improved transparency, accessible information, and robust regulatory frameworks.Effective solutions demand a shift from reactive to proactive measures.
By proactively identifying and addressing potential risks, the AI development community can build trust and foster a more responsible and ethical approach to AI development and deployment. This proactive approach not only safeguards users but also ensures that AI systems are developed and utilized responsibly.
Strategies for Improving Transparency
Transparency is fundamental to building trust in AI systems. Clear communication of potential risks and limitations associated with AI algorithms is essential. This involves providing detailed explanations of how AI systems work, including their limitations, potential biases, and areas of uncertainty. Companies must proactively disclose any known safety concerns, rather than waiting for users to discover them.
User-friendly interfaces that explain AI decisions are crucial, making the technology more accessible and understandable.
Accessible AI Safety Information
Making AI safety information readily available and accessible to all stakeholders is critical. This involves translating complex technical information into plain language, creating easily digestible summaries, and utilizing multiple channels to disseminate this information. Educational resources, workshops, and online platforms can facilitate widespread knowledge of AI safety guidelines and best practices.
Implementing Solutions to Mitigate Risks
Several practical solutions can be implemented to mitigate the risks associated with hidden AI safety warnings. These include:
- Establishing standardized safety protocols: Creating industry-wide standards for AI safety testing and evaluation can ensure consistency and a baseline level of safety across different AI systems. This will require collaboration between researchers, developers, and regulatory bodies to establish universally recognized standards for AI system safety and security. Examples include clear guidelines for data privacy, bias detection, and mitigation strategies.
- Developing comprehensive AI safety checklists: Creating detailed checklists that cover various aspects of AI system development, from data collection and preprocessing to model training and deployment, can help developers proactively identify potential risks. These checklists should cover areas like data quality, bias detection, potential vulnerabilities, and post-deployment monitoring strategies. This systematic approach would significantly improve the detection and prevention of hidden risks.
- Establishing independent review boards: Forming independent review boards composed of experts in AI safety, ethics, and relevant fields can provide a critical assessment of AI systems before deployment. This will ensure rigorous scrutiny and identification of potential safety concerns, ensuring that all stakeholders are aware of any limitations or risks.
Regulatory Frameworks for Prevention
Robust regulatory frameworks can play a crucial role in preventing hidden AI safety warnings. These frameworks should mandate specific disclosures, establish penalties for non-compliance, and encourage transparency and accountability. Regulations should address the specific needs of different AI systems and their applications, considering the diverse contexts in which AI is used.
Comparative Analysis of Solutions
Solution | Description | Advantages | Disadvantages |
---|---|---|---|
Establishing standardized safety protocols | Industry-wide standards for AI safety testing and evaluation. | Ensures consistency, baseline safety, and collaboration. | May be slow to adapt to emerging technologies, could face resistance from companies. |
Developing comprehensive AI safety checklists | Detailed checklists for AI system development. | Proactive risk identification, systematic approach. | May not cover all potential risks, requires ongoing updates. |
Establishing independent review boards | Expert review of AI systems before deployment. | Rigorous scrutiny, identification of potential concerns. | Can be costly and time-consuming, potential for bias in the review process. |
Outcome Summary
In conclusion, DeepSeeks hidden AI safety warnings present a significant threat to the integrity and safety of AI systems. Understanding the motivations, methods, and potential consequences of hidden warnings is crucial for developing safer AI. This discussion emphasizes the importance of transparency and proactive measures to prevent hidden risks and promotes a deeper understanding of the need for explicit and readily accessible AI safety information.
The case studies and potential solutions offered provide actionable insights for improving AI safety practices.