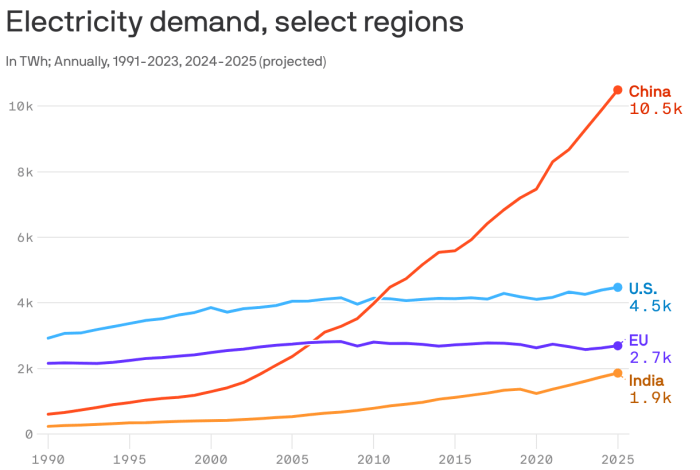
AI rising global electricity demand is a complex issue with far-reaching implications. From powering data centers to optimizing energy grids, AI is transforming how we use energy. This exploration dives into the intricate relationship between artificial intelligence and global electricity consumption, examining its impact on various industries and the potential solutions for managing this growing need.
This post will delve into the different ways AI technologies are driving up global electricity demand. We’ll explore how various industries, from manufacturing to cloud computing, are using AI and the impact it has on their power consumption. We’ll also examine potential solutions and the future of energy systems in the age of artificial intelligence.
AI and Electricity Consumption
Artificial intelligence (AI) is rapidly transforming various sectors, and its impact on energy consumption patterns is undeniable. From data centers powering complex algorithms to smart grids managing electricity distribution, AI is weaving itself into the fabric of our energy systems. This intricate relationship is driving both increased demand and the potential for unprecedented efficiency. Understanding this evolving dynamic is crucial to anticipating future energy needs and developing sustainable solutions.AI’s influence on electricity consumption stems from its ability to optimize processes and predict future needs.
This allows for more efficient resource allocation and a reduction in waste, but also necessitates a greater overall energy capacity. Historically, technological advancements have always correlated with shifts in energy consumption, and AI is no exception. The increasing complexity and sophistication of AI systems require substantial computing power, often housed in large-scale data centers, thus driving up electricity demand.
AI’s growing demand for energy is a real concern, with its complex algorithms consuming vast amounts of power. This computational intensity often makes it difficult to understand exactly how these systems arrive at their conclusions, much like a “black box” definition of black box. The lack of transparency in AI’s decision-making processes further complicates predicting and managing the rising global electricity needs brought about by this technology.
AI Applications Increasing Electricity Demand
The growth of AI applications is directly contributing to a rise in global electricity demand. These applications encompass a wide range of sectors, demanding more energy to perform their tasks. Examples include:
- Machine Learning Training: The training of large language models and other complex machine learning models requires massive amounts of computational power. This translates to substantial energy consumption within data centers housing these systems. For instance, training a single large language model can consume the equivalent of hundreds of thousands of homes’ annual electricity use.
- Autonomous Vehicles: The sophisticated sensor systems and processing power needed for self-driving cars directly translate to increased electricity consumption. The computing requirements for real-time data processing and decision-making significantly contribute to the energy demands of this sector.
- AI-Powered Robotics: Industrial robots using AI for tasks like quality control and assembly operations require substantial energy input. The computational demands and power needs of these systems contribute directly to overall electricity usage.
AI’s Potential for Energy Optimization
Despite increasing demand, AI also presents a powerful tool for optimizing energy use. This involves sophisticated algorithms that can predict energy consumption patterns, optimize energy distribution, and integrate renewable energy sources. The following examples highlight the potential:
- Smart Grid Management: AI algorithms can analyze real-time data from electricity grids, allowing for more efficient distribution and demand response programs. This reduces energy waste and improves the overall efficiency of power systems.
- Predictive Maintenance: AI can analyze data from power plants and other infrastructure to predict equipment failures, preventing costly downtime and optimizing maintenance schedules. This directly translates into less wasted energy.
- Energy-Efficient Data Centers: AI can optimize cooling systems, power allocation, and resource utilization within data centers, leading to significant energy savings.
Historical Context of Energy Consumption
The relationship between technological advancements and energy consumption has been a recurring theme throughout history. Each new era of technological innovation has brought about a corresponding shift in energy consumption patterns. This is not a new phenomenon, as earlier technological advancements have also led to similar trends.
- The Industrial Revolution: The advent of steam engines and factories marked a significant increase in energy demand. This highlights the strong correlation between technological shifts and energy consumption.
- The Rise of the Automobile: The increasing use of automobiles, requiring gasoline or electricity, also increased overall energy demand.
- The Digital Age: The proliferation of computers and the internet has drastically increased the need for electricity, further solidifying this trend.
AI-Driven Industries and Electricity Demand
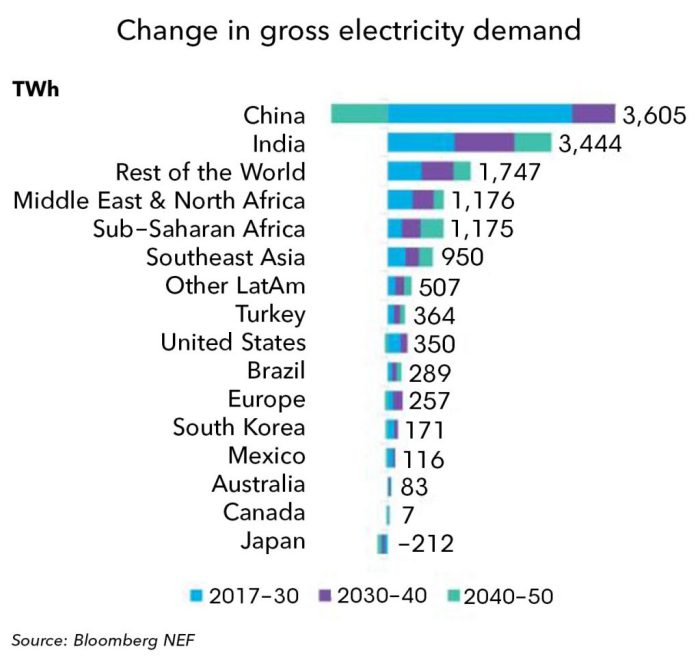
Artificial intelligence (AI) is rapidly transforming various industries, leading to increased automation and efficiency. This surge in AI adoption, however, directly correlates with a significant rise in electricity consumption. Understanding the impact of AI on different sectors is crucial for forecasting future energy needs and developing sustainable solutions. This analysis explores the electricity demand implications of AI in key industries.
Major Industries Impacted by AI
Several industries are experiencing substantial transformations due to AI integration. These include manufacturing, transportation, and data centers. The implementation of AI-powered systems in these sectors often leads to increased automation and data processing, thus requiring more energy.
Manufacturing
AI-powered automation in manufacturing significantly impacts electricity consumption. Automated robots, predictive maintenance systems, and AI-driven quality control processes require substantial computational power and data processing, leading to higher energy demands. For instance, sophisticated AI algorithms for optimizing production lines may necessitate specialized servers and powerful processing units.
Transportation
The introduction of AI in transportation, particularly in autonomous vehicles and logistics, has a notable impact on electricity consumption. Self-driving cars, trucks, and delivery systems rely on sophisticated sensors, computing power, and communication networks, which all contribute to a substantial increase in energy requirements. Electric vehicle charging infrastructure, which needs significant electricity capacity, is also a major factor.
Data Centers
Data centers are crucial for AI operations, hosting the servers and networks that drive AI applications. The massive data processing required for AI tasks leads to substantial energy consumption in these facilities. The continuous operation and cooling needs of data centers contribute significantly to overall energy demands.
Comparison of Electricity Consumption Profiles
Pre-AI, electricity consumption in these sectors was typically lower due to manual labor and less complex processes. AI implementation often leads to 24/7 operations, with more demanding processing needs and significant cooling requirements for servers and equipment. This shift to continuous operation and data processing results in a higher electricity consumption profile compared to the past.
Specific AI Applications Affecting Power Usage
Numerous AI applications directly affect electricity usage. For instance, deep learning models used for image recognition or natural language processing in manufacturing or transportation require considerable computational resources. AI-driven predictive maintenance models in manufacturing plants analyze sensor data, requiring real-time processing and transmission, impacting power consumption.
Electricity Consumption Table
AI-Related Sector | Estimated Electricity Consumption (kWh/year) | Description |
---|---|---|
Manufacturing (AI-powered automation) | 10,000,000 – 50,000,000 | Automated robots, predictive maintenance, quality control |
Transportation (autonomous vehicles) | 500,000 – 5,000,000 | Self-driving cars, trucks, and delivery systems |
Data Centers (AI processing) | 100,000,000 – 1,000,000,000 | Hosting servers and networks for AI applications |
Note: The electricity consumption figures are estimates and can vary significantly depending on factors such as specific AI applications, scale of operations, and energy efficiency measures.
AI’s Role in Data Centers and Cloud Computing
The explosive growth of data centers and cloud computing is inextricably linked to the rise of AI. As AI algorithms become more complex and data volumes swell, the need for powerful computing resources intensifies, pushing the boundaries of data center infrastructure and demanding more energy. This dependence on robust computing power fuels a continuous cycle of innovation and infrastructure expansion, further highlighting the intricate relationship between AI, data centers, and energy consumption.AI is not merely a consumer of energy in data centers; it’s also a powerful tool for optimizing energy usage.
By automating tasks, analyzing vast datasets, and predicting future needs, AI is revolutionizing how data centers operate, leading to significant improvements in energy efficiency. However, the very complexity and sophistication of these AI-driven systems often present new challenges in managing and optimizing energy consumption.
AI Accelerating Data Center and Cloud Growth
AI is driving the expansion of data centers and cloud computing through several key mechanisms. The need for ever-increasing processing power for complex AI models demands larger and more sophisticated data centers. Cloud providers are continuously scaling their infrastructure to accommodate this rising demand, leading to a substantial increase in electricity consumption. Furthermore, AI-powered tools and applications themselves rely heavily on cloud computing platforms for their operation and data storage.
This interdependency fuels a positive feedback loop, where AI-driven demand continuously drives cloud infrastructure growth, and in turn, increased energy consumption. For example, the training of large language models requires enormous computational resources, resulting in substantial energy expenditures. This trend is expected to continue as AI models become more intricate and powerful.
Energy Efficiency Strategies in Data Centers
Data centers employ various strategies to improve energy efficiency. These include optimizing server placement for optimal cooling, implementing highly efficient cooling systems like liquid cooling, and using advanced power management technologies to reduce energy waste. However, these strategies have inherent limitations. For example, while liquid cooling offers significant potential for reducing energy consumption in data centers, the cost of implementation and maintenance can be substantial.
Furthermore, the complexity of these systems can introduce new points of failure. Another approach involves server virtualization, allowing multiple virtual machines to run on a single physical server, thus potentially reducing energy consumption by reducing the number of physical servers needed. Yet, virtualization can introduce its own complexity and potential performance bottlenecks.
AI for Data Center Operations and Power Consumption
AI is playing a critical role in managing and optimizing data center operations, thereby impacting power consumption. AI algorithms can predict energy demands, optimize cooling systems, and automate maintenance tasks, leading to substantial energy savings. For instance, AI-powered predictive maintenance can identify potential equipment failures before they occur, enabling proactive repairs and preventing unexpected downtime, thereby minimizing energy consumption during the repair process.
AI-driven load balancing techniques ensure that servers are utilized efficiently, preventing underutilization or overutilization that leads to unnecessary energy consumption.
Comparison of Energy Management Approaches
Approach | Description | Advantages | Limitations |
---|---|---|---|
Liquid Cooling | Utilizes liquid instead of air to cool servers | Potentially significant energy savings | High initial investment, complexity of maintenance |
Server Virtualization | Multiple virtual machines on a single physical server | Reduced number of physical servers, potential energy savings | Potential performance bottlenecks, complexity of management |
AI-driven Predictive Maintenance | Using AI to predict equipment failures | Proactive repairs, prevention of unexpected downtime | Requires substantial data for training, potential for errors in predictions |
This table highlights the different strategies employed to manage energy in data centers, showcasing their respective advantages and disadvantages. Each approach presents a unique set of trade-offs that must be carefully considered in the context of specific data center needs and resources. The use of AI in these areas is rapidly evolving, and further advancements are expected in the future.
AI’s rising global electricity demand is a serious concern, especially when considering the complex web of interconnected systems. The rapid advancements in AI are pushing the limits of our current infrastructure, and this increased energy consumption could have unforeseen consequences. This mirrors the potential dangers of neglecting the foundational principles of the world Franklin D. Roosevelt helped build, which could be explored further in this insightful piece on the perils of jettisoning the world Franklin Roosevelt created.
Ultimately, as AI continues to evolve, careful consideration of its energy footprint is crucial to ensure a sustainable future.
AI and the Future of Energy Systems: Ai Rising Global Electricity Demand
Artificial intelligence (AI) is poised to revolutionize energy systems, enabling smarter grids, optimized renewable energy integration, and a more sustainable future. The increasing complexity of energy production and consumption demands innovative solutions, and AI offers a powerful toolset to address these challenges. From predicting energy demand to optimizing renewable energy sources, AI is rapidly transforming the landscape of global energy management.AI’s potential in optimizing energy systems extends beyond simply improving efficiency.
It can foster a more resilient and adaptable energy infrastructure, capable of handling fluctuating renewable energy generation and accommodating evolving consumption patterns. This, in turn, can lead to significant cost savings and a more reliable energy supply for consumers.
AI-Powered Smarter Energy Grids
AI algorithms can analyze vast amounts of data from various sources, including weather patterns, energy consumption trends, and grid performance metrics. This data analysis allows for real-time adjustments to energy distribution, preventing outages and optimizing energy flow. Predictive maintenance, powered by AI, can anticipate equipment failures in power plants and transmission lines, allowing for proactive repairs and minimizing disruptions.
This proactive approach is crucial in maintaining grid reliability, a key factor in the sustainability and stability of the energy system.
Optimizing Renewable Energy Integration
The integration of renewable energy sources, such as solar and wind power, is critical for a sustainable energy future. AI can play a pivotal role in optimizing the output of these intermittent sources. By analyzing weather forecasts and historical data, AI can predict the generation capacity of solar and wind farms, enabling grid operators to balance supply and demand effectively.
This integration requires sophisticated algorithms that can account for the inherent variability of renewable energy generation, ensuring a reliable and consistent energy supply. Furthermore, AI can optimize energy storage systems to accommodate fluctuations in renewable energy production, creating a more stable and efficient energy grid.
Emerging Technologies Combining AI and Renewable Energy
Several emerging technologies are combining AI and renewable energy to create innovative solutions. For example, AI-powered microgrids can manage localized energy generation and consumption, allowing communities to become more self-sufficient. These microgrids are particularly useful in remote areas or during power outages, ensuring continued access to essential services. Furthermore, AI-driven smart meters can provide detailed insights into energy consumption patterns, helping consumers make informed decisions about energy usage and reducing waste.
Future Impact on Energy Source Demand
AI’s impact on energy source demand is complex and multifaceted. While AI can optimize the use of existing fossil fuel power plants, it also plays a crucial role in accelerating the transition towards renewable energy sources. AI can analyze the cost-effectiveness of different energy sources, taking into account factors such as fuel prices, environmental regulations, and technological advancements. This analysis will likely drive a shift towards renewable energy sources, as their cost competitiveness improves and technological advancements reduce the variability in their generation.
This transition will be gradual, but AI-powered optimization will be a key factor in shaping the future energy mix.
Global Implications of AI-Driven Electricity Demand
The rise of artificial intelligence (AI) is undeniably transforming industries, and this transformation is placing unprecedented pressure on global electricity grids. As AI-powered systems become more ubiquitous, the demand for electricity is expected to surge, presenting both opportunities and challenges for various regions and countries. Understanding these implications is crucial for policymakers and businesses alike to adapt and mitigate potential risks.The increasing adoption of AI in diverse sectors, from data centers to manufacturing, is directly correlated with a growing need for power.
This escalating demand will not be evenly distributed across the globe, leading to unique challenges and opportunities in different regions. Examining the underlying factors and potential consequences of this global trend is essential to navigating the future of energy and AI.
Electricity Consumption Trends Across Regions
Different regions exhibit distinct electricity consumption patterns. Developed nations often have higher per capita electricity consumption due to factors such as advanced industrialization and higher standards of living. Developing countries, while experiencing rapid industrialization and urbanization, typically exhibit lower per capita consumption. Analyzing these trends is vital to understanding the future energy demands of different regions and to anticipate potential strains on infrastructure.
- North America, with its advanced technological infrastructure, generally exhibits high per capita consumption. The United States, for example, consumes significantly more electricity than many developing countries. This high demand is largely attributed to a mix of factors, including extensive industrial activity, reliance on energy-intensive technologies, and the prevalence of energy-hungry devices and appliances in households.
- Europe, known for its advanced manufacturing and technological sectors, also experiences high electricity consumption. However, European nations often implement stricter environmental regulations and energy efficiency standards compared to other regions. This helps to temper consumption growth, though significant demand remains.
- Asia, a region experiencing rapid industrialization and urbanization, demonstrates a significant increase in electricity consumption. China and India, in particular, are experiencing exponential growth in energy demand, largely driven by industrialization and a rising middle class. This trend poses a challenge for sustainable energy development.
- Africa, with its diverse economies and varying levels of industrialization, shows a wide range of consumption patterns. While some African nations are experiencing substantial growth in electricity consumption, others are facing considerable challenges in expanding access to electricity.
Potential Challenges and Opportunities
The increasing electricity demand, driven by AI, presents a complex mix of challenges and opportunities. Successfully navigating this transition hinges on a careful assessment of these factors and the implementation of appropriate strategies.
- Infrastructure Limitations: Existing electricity grids may struggle to accommodate the surge in demand, potentially leading to blackouts or service interruptions. Upgrading infrastructure and investing in renewable energy sources are crucial to ensuring reliable power supply.
- Energy Security: Over-reliance on specific energy sources can create vulnerabilities. Diversifying energy portfolios and promoting energy independence can help to mitigate risks associated with supply disruptions.
- Environmental Impact: Increased electricity demand can exacerbate environmental concerns, particularly if the energy mix is not sufficiently sustainable. Promoting renewable energy sources and improving energy efficiency are essential to minimizing the environmental footprint.
- Economic Growth: The growth of AI-driven industries can create new jobs and economic opportunities. However, a careful approach to investment and infrastructure development is essential to avoid widening economic disparities.
Factors Contributing to Varying Consumption Rates, Ai rising global electricity demand
Several factors contribute to the disparity in electricity consumption rates across nations. These factors, often intertwined, include economic development, industrialization, population density, technological advancements, and energy efficiency standards.
AI’s growing demand for energy is causing a surge in global electricity needs, which is a complex issue. It’s interesting to consider how this parallels the ripple effects of things like trump tariffs, stock buyouts, and alleged insider trading and market manipulation, as discussed in this article trump tariffs stock buy djt tip insider trading market manipulation.
Ultimately, these interconnected economic forces, including the escalating AI energy demands, will shape our future energy landscape.
- Economic Development: Countries with more advanced economies and higher GDPs tend to consume more electricity due to the prevalence of industrial activities and energy-intensive consumer goods.
- Industrialization: Industrial sectors are typically large consumers of electricity. The level of industrialization significantly impacts a nation’s overall energy demand.
- Population Density: Higher population density often leads to greater energy demand for residential and commercial purposes.
- Technological Advancements: Technological advancements can both increase and decrease energy consumption. While new technologies often require more power, advancements in energy efficiency can mitigate this demand.
- Energy Efficiency Standards: Countries with stricter energy efficiency regulations often exhibit lower electricity consumption rates, as consumers and industries are incentivized to adopt more energy-efficient practices.
Economic and Social Impacts
The rise in electricity demand has significant economic and social implications. These implications are multifaceted and range from the creation of new industries and jobs to the potential for increased energy costs and social inequality.
- Economic Opportunities: The growth of AI industries creates opportunities for job creation in research, development, and implementation of AI-related solutions.
- Economic Challenges: Increased energy costs can impact various sectors, potentially impacting consumer prices and economic competitiveness.
- Social Equity: Uneven access to electricity and related resources can exacerbate social inequalities, highlighting the need for equitable energy policies.
Addressing the Challenges of Increasing Demand
The surge in global electricity demand, fueled by the burgeoning AI sector, presents a significant challenge to the sustainability of our energy systems. Meeting this demand while minimizing environmental impact requires a multifaceted approach, encompassing technological advancements, policy changes, and a shift in societal perspectives. Strategies that focus on energy efficiency and renewable energy sources are crucial to mitigate the negative effects of this increased consumption.The increasing reliance on AI across industries necessitates a corresponding focus on sustainable energy practices.
This requires innovative solutions to manage the energy needs of AI-powered systems and data centers, as well as developing more energy-efficient AI algorithms and applications. By proactively addressing this challenge, we can ensure a future where AI’s transformative potential is realized without jeopardizing the planet’s resources.
Potential Solutions to Address Rising Demand
Several strategies can mitigate the impact of escalating AI-driven electricity consumption. These include improving energy efficiency in AI technologies, implementing sustainable energy policies, and promoting responsible AI development practices.
- Developing Energy-Efficient AI Technologies: The design and implementation of AI algorithms with lower energy footprints are essential. This includes optimizing machine learning models for reduced computational complexity, employing hardware architectures tailored for energy efficiency, and exploring novel approaches like neuromorphic computing. For example, using specialized chips designed for AI tasks can significantly reduce power consumption compared to general-purpose processors.
- Promoting Sustainable Energy Policies: Governments and regulatory bodies can play a vital role in encouraging the adoption of energy-efficient AI practices. Policies that incentivize the development and deployment of energy-efficient AI technologies and infrastructure, coupled with regulations that address the energy footprint of data centers and cloud computing, are necessary. This includes offering tax credits for companies implementing energy-efficient AI solutions, setting standards for energy consumption in data centers, and encouraging the use of renewable energy sources in AI-related infrastructure.
- Enhancing Energy Efficiency in AI-Driven Systems: Strategies to enhance energy efficiency in AI-driven systems are diverse and crucial. These include optimizing algorithms, utilizing more efficient hardware, and adopting cloud computing approaches to distribute the workload across multiple servers. For example, using serverless computing architectures can further reduce energy consumption by dynamically scaling resources based on demand.
Strategies for Enhancing Energy Efficiency in AI-Driven Systems
Different strategies exist to optimize energy consumption within AI systems. These strategies aim to minimize the environmental impact of these systems.
Strategy | Description | Impact |
---|---|---|
Algorithm Optimization | Improving the efficiency of AI algorithms by reducing the number of computations and utilizing optimized data structures. | Directly reduces energy consumption by lowering the computational load. |
Hardware Optimization | Utilizing specialized hardware designed for AI tasks, such as GPUs and TPUs, that are more energy-efficient than general-purpose CPUs. | Significant reduction in energy consumption compared to traditional hardware. |
Cloud Computing Approaches | Distributing the workload across multiple servers in a cloud environment, allowing for dynamic resource allocation and load balancing. | Optimizes energy usage by dynamically scaling resources and reducing idle power consumption. |
Case Studies of AI and Energy
AI is rapidly transforming energy systems, and real-world applications are demonstrating significant potential for efficiency gains. By leveraging data analysis and machine learning, AI can optimize energy consumption, predict demand fluctuations, and automate maintenance tasks. These advancements promise to be crucial in addressing the rising global demand for electricity.
AI in Manufacturing for Energy Efficiency
AI-powered predictive maintenance is revolutionizing manufacturing sectors, leading to substantial energy savings. By analyzing sensor data from machinery, AI algorithms can identify potential equipment failures well in advance, preventing costly downtime and optimizing maintenance schedules. This proactive approach reduces energy consumption during maintenance procedures and extends the lifespan of critical infrastructure. For example, a steel mill using AI-driven predictive maintenance reduced energy consumption by 15% by preventing unplanned equipment outages.
Smart Grids and AI-Driven Demand Response
Smart grids, coupled with AI, are enabling real-time adjustments to energy consumption. AI algorithms can analyze energy consumption patterns, predict future demand, and automatically trigger demand response programs. This capability allows utilities to manage peak loads effectively, reduce reliance on fossil fuels, and increase the use of renewable energy sources. A smart grid in California, utilizing AI, successfully reduced peak demand by 10% during heat waves, avoiding the need for costly power plant upgrades.
AI-Optimized Building Management Systems
AI is transforming building management systems (BMS), leading to significant energy savings in commercial and residential spaces. By analyzing real-time data from various sensors, AI algorithms can optimize lighting, heating, and cooling systems. This automation adjusts parameters dynamically, ensuring comfort while minimizing energy waste. A large office complex in New York City implemented an AI-powered BMS, reducing energy consumption by 20% and improving employee comfort levels.
Table: Case Studies of AI and Energy Efficiency
Sector | AI Application | Outcome | Lessons Learned |
---|---|---|---|
Manufacturing (Steel Mill) | AI-driven predictive maintenance | 15% reduction in energy consumption, reduced downtime | Proactive maintenance significantly impacts energy efficiency. |
Utilities (California Smart Grid) | AI-powered demand response | 10% reduction in peak demand during heat waves | AI enables efficient management of energy demand. |
Buildings (Office Complex) | AI-optimized BMS | 20% reduction in energy consumption, improved employee comfort | AI-powered optimization of building systems leads to significant savings. |
Ultimate Conclusion
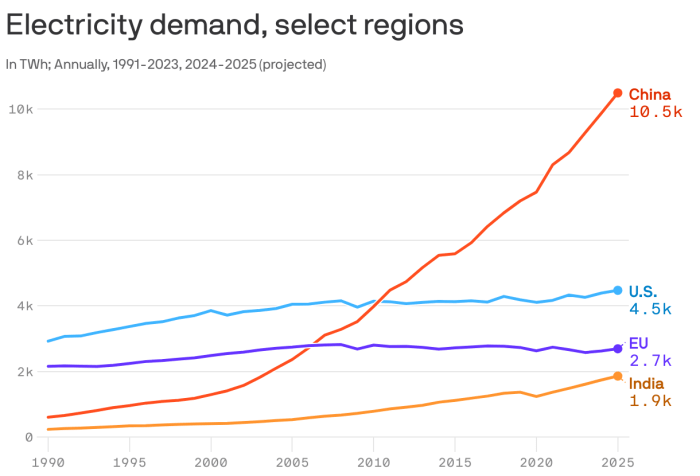
In conclusion, the rise of AI is undeniably increasing global electricity demand. While this presents challenges, it also unlocks opportunities for innovation in energy efficiency and the development of smarter energy grids. The future hinges on our ability to harness AI’s potential while mitigating its impact on our energy systems. By embracing sustainable practices and fostering research in energy-efficient AI, we can navigate this transition effectively and create a more sustainable energy future.